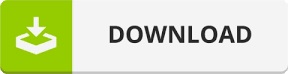
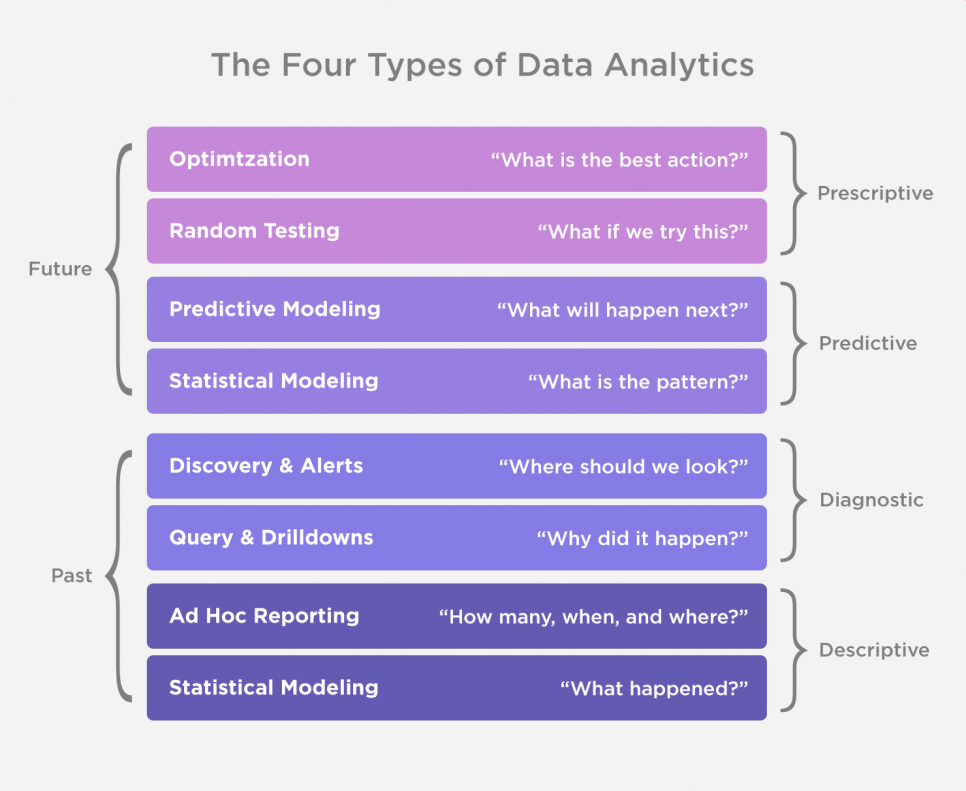
By the end of the book, you will have the practical knowledge to tackle real data problems in your organization or daily life. The book includes practical examples, visual examples, and two bonus coding exercises in Python.
#Basic data analytics methods using r how to#
How to make better business decisions using Data Visualization and Business Intelligence When and how to use Regression Analysis, Classification, Clustering, Association Analysis, and Natural Language Processing How to explain the distinction between Data Mining, Machine Learning, and Analytics to your colleagues New trends in data analytics, including what is Alternative Data and why not many people know about it Where to store your data, including Big Data How to recognize the common data types every data scientist needs to master

Here's what you will learn from reading the book: This book teaches you the vocabulary, tools, and basic algorithms to think like a data scientist. You will make better decisions with this easy deconstructed guide to data analytics. The book is a must-read for anyone looking to embark on a lifelong journey toward analytical enlightenment.ĭata Analytics for Absolute Beginners: A Deconstructed Guide to Data Literacy by Oliver Theobald gives an introduction to Data, Data Visualization, Business Intelligence, and Machine Learning. Readers will enjoy an easy-to-understand piece that squeezes in a wide range of data analytics topics in a systematic way. Some common analytical and statistical packages in R
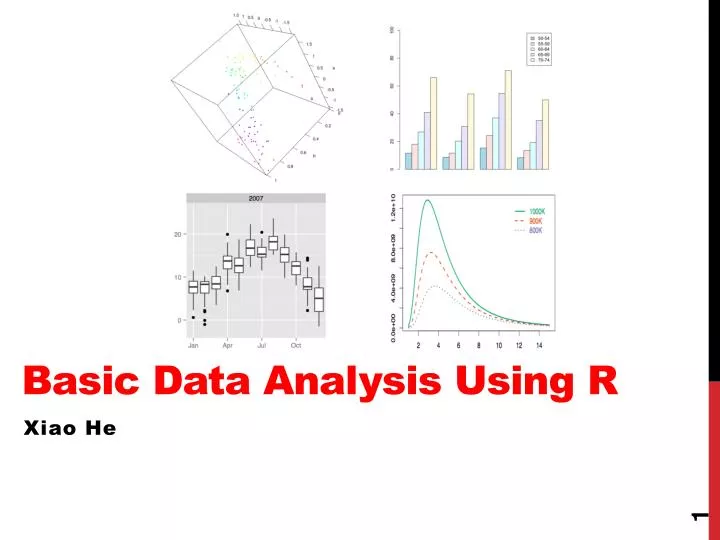
Some machine learning approaches to data analytics Unraveling probability distributions and inferential statistics processes The essentials of machine learning and how it relates to data analytics The two common data structures employed in data analytics This book will help you uncover the nitty-gritty of data and big data. You will find useful strategies and resources that can be used to derive reasonable insight into the data generated. Benjamin Smith is a beginner-friendly guide that presents the A-Z on the realms of data analytics. Data analytics gives you the perfect opportunity to become a well-paid freelancer or consultant.ĭata Analytics: A Comprehensive Beginner’s Guide by Mr. It represents perfect freelancing opportunities: Everyone is looking for ways to diversify their sources of income. Increasing pay for data analytics professionals: Data analytics professionals are getting paid more and more and this trend is evident across the globe. There is an increasing number of job postings looking for data analysts and consultants. Increasing job opportunities: Organizations are looking for specialists who can comprehensively gather, interpret and use data. There is no industry or sector which has remained untouched from the reach of Data Analytics. Īnalytics is everywhere: The use of data analytics professionals to foster growth is slowly catching on and is becoming more important.High demand: Data analytics is now a priority for top organizations. Let's have a look at the reasons why you should learn data analytics. With such a boom in the use of analytics, these skills are the way of the future. For this, commands such as cor() can be used.With data becoming the new oil, Data Analysts are more in need than ever. Relationships among Features: You may want to understand relationship that exists between different features.Visualize Relationships & Data Patterns:You could one or more plots such as hist(), plot etc to identify the data pattern and also, relationship between different features.This procedure is also termed as holdout method. The training dataset is used to generate the model, which is then applied to the test dataset to generate predictions for evaluation. Training & Test DatasetsFor preparing data for actual analysis, one may want to identify the sample datasets for training and test purpose.For nominal data, command such as table() comes very handy. Observe Data Observations & Features:Commands such as summary() comes very handy to explore the spread of the data across different features.Studying Data FrameStudy the data frame exploring different observations and features.Exploring Data Types:Identify which data is numeric and which of them are nominal (stringsAsFactors option) and import the data appropriately.Data Loading:Load the data into the tool.Following are some of the steps you would want to perform in this step: The next step is to explore the data and prepare it for further analysis.
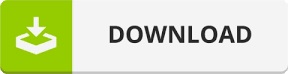